41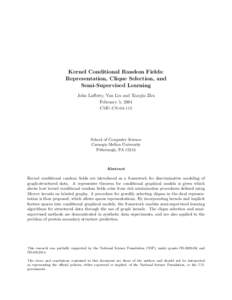 | Add to Reading ListSource URL: www.aladdin.cs.cmu.edu- Date: 2010-11-17 10:54:43
|
---|
42 | Add to Reading ListSource URL: jmlr.org- Date: 2014-02-16 19:30:21
|
---|
43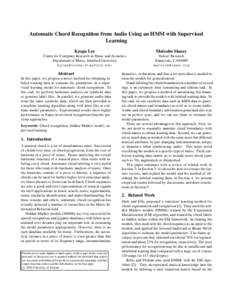 | Add to Reading ListSource URL: ccrma.stanford.edu- Date: 2006-09-09 18:08:35
|
---|
44 | Add to Reading ListSource URL: www.flosko.at- Date: 2016-10-01 10:24:48
|
---|
45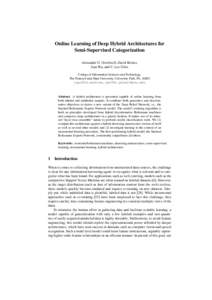 | Add to Reading ListSource URL: www.david-reitter.com- Date: 2016-12-01 11:37:10
|
---|
46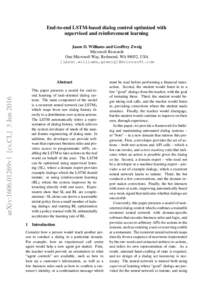 | Add to Reading ListSource URL: arxiv.org- Date: 2016-06-06 20:40:38
|
---|
47 | Add to Reading ListSource URL: math.nyu.edu- Date: 2006-11-05 17:29:36
|
---|
48 | Add to Reading ListSource URL: www.isical.ac.in- Date: 2014-12-05 23:51:05
|
---|
49 | Add to Reading ListSource URL: www.ilhaire.eu- Date: 2013-10-03 05:33:46
|
---|
50 | Add to Reading ListSource URL: aml.media.mit.edu- Date: 2011-11-03 19:34:09
|
---|